Prototype as of September 2019
Street Sense Features:
- Measurement sensing:
- ozone, in ppb
- NO2, in ppb
- PM2.5 particulates
- sound level, in dBA
- continuous audio recording for post analysis
- temperature and humidity
- Measurement recording
- on-board micro SD Card media
- continuous recording of audio stream to WAV file
- air quality measurements logged to files
- SD Card removable to enable file download
- recorded data is time-stamped
- Power
- battery or USB powered
- minimum 24 hours operation time on battery
- USB rechargeable without battery removal
- Enclosure
- weatherproof
- simple attachment to a street level structure such as a lighting pole
- allows air flow thru
- Connectivity
- WiFi for pushing sensor data to an internet cloud database
- User Interface
- on-board display to view readings and verify operation
- simple operation requiring minimal training
- 100% Open
- Open Hardware - schematics, BOMs, etc
- Open Software - written in MicroPython - all code hosted on public github account
Stretch ambitions:
- open API to allow customization using MicroPython
Project Parts Budget: USD $225
Hardware building blocks
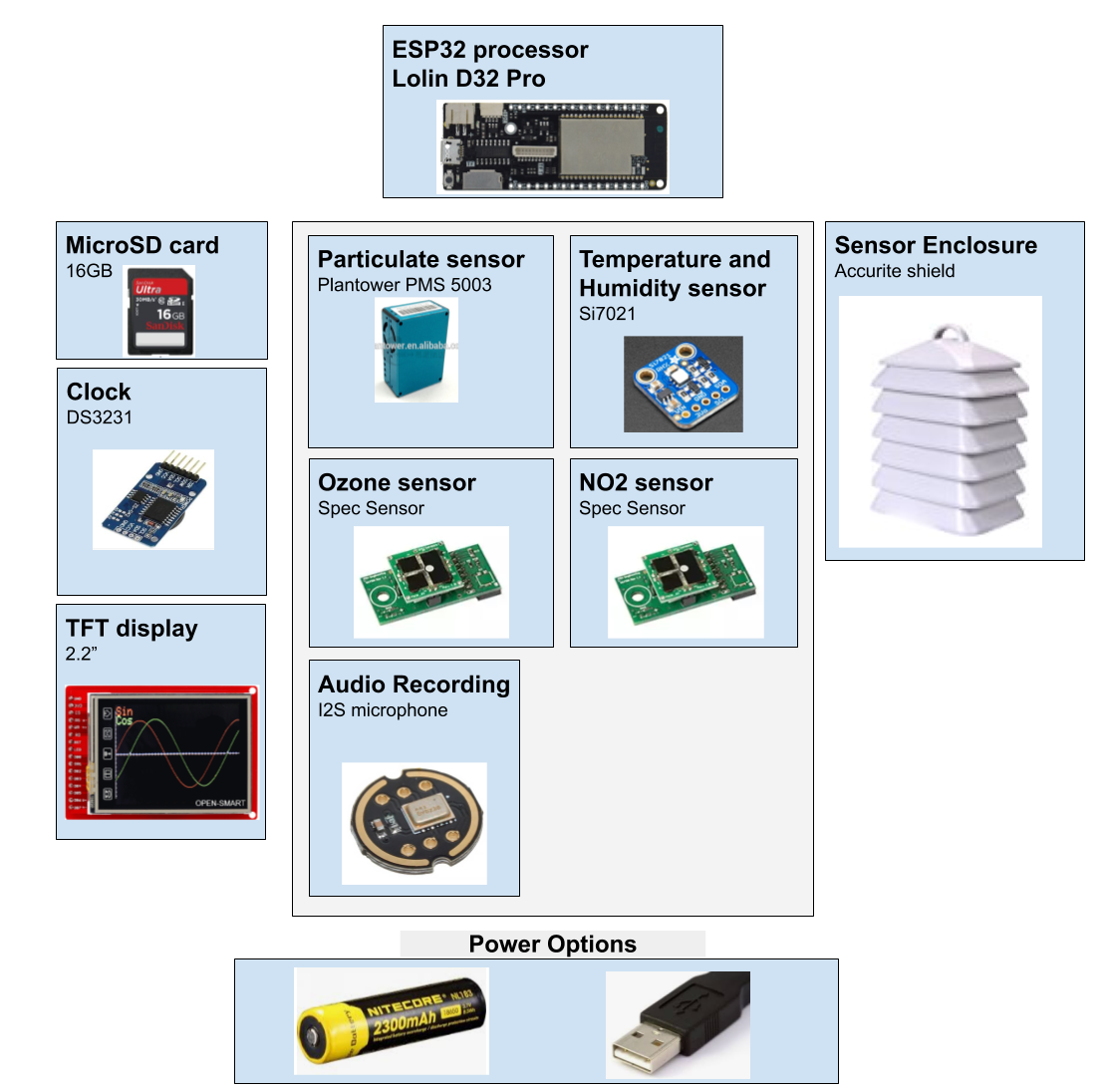
"Need Help" Section:
This section describes areas where I am having difficulties and could use some help from Hackaday experts. Please consider commenting if you have ideas on any of these problems. thanks!
April 2019:
1. Oscillations from Spec Sensor ozone and NO2 sensors. I think it is caused by noise in the 3.3V rail power supplied to both the sensors and ADC. The initial breadboard proto no doubt contributes to this problem. Described in this project log. Any ideas appreciated! e.g. LC filtering to create cleaner analog power rail? Is it worth trying to make a soldered proto? Or, is a custom PCB the only way to improve this?
2. SDCard write delays. The 16GB card that I am using (SanDisk Ultra 16GB) claims to be a class 10 device. But, some 512Byte sector writes take a really long time...e.g. >50ms. And, there can be multiple back-to-back writes > 50ms. These long SDCard write block the waiting coroutines in the cooperative multitasking asyncio design. My main need is fast writes. Fast reads don't matter for this application. Are there better choices for SD Cards that support fast writes?
hello Mike
congratulations, yours is a great project!
Should I read the python values from the OZONO SPEC sensor directly on the raspberry, could you tell me if there is a specific library for SPEC or if your micropython would already work?
Can you say the SPEC OZONO sensor code used, is it a digital or analog sensor?
thanks for now